Scientists look to the skies to improve crop predictions on earth
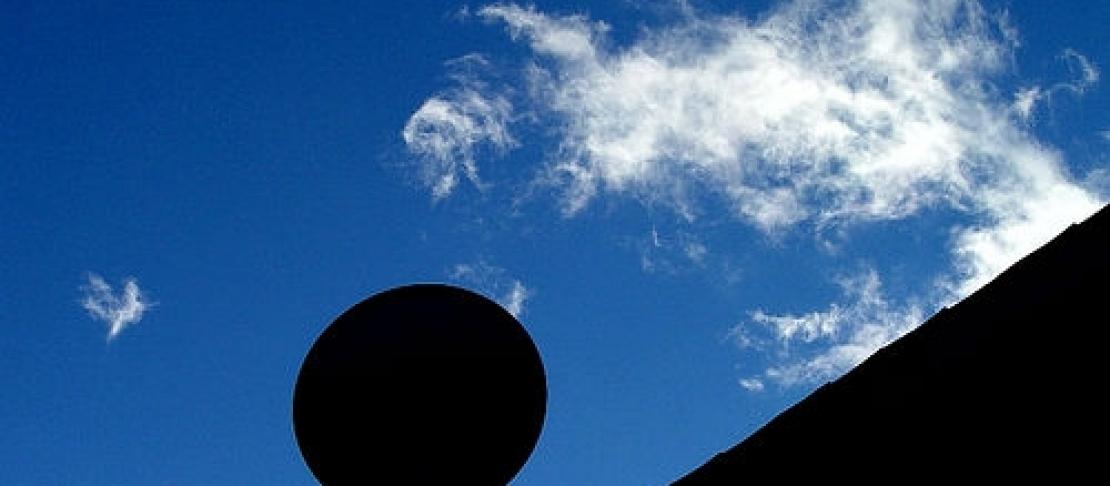
by Alexa Jay and Amor Ines
Crop yield predictions made during the growing season are relevant to many agricultural and food security decisions, including food safety net and relief programs, agricultural insurance, and management of agricultural inputs and credit supplies. The CGIAR Research Program on Climate Change, Agriculture, and Food Security (CCAFS) Theme 2: Adaptation Through Managing Climate Risk supports advances in crop forecasting in service of its goal to enhance the resilience of rural livelihoods and food systems to climate-related risk.
A joint CCAFS Theme 2 project between the International Research Institute for Climate and Society at Columbia University (IRI) and NASA’s Jet Propulsion Laboratory (JPL) is investigating the use of satellite data to improve the accuracy of crop yield forecasting. Promising results in homogenous, large-scale farming environments indicate that incorporation of remotely sensed information on vegetation cover and soil moisture into crop models can reduce errors in yield predictions. Research is ongoing to adapt these techniques for smallholder farming environments, where plots are typically smaller than the pixel size of available remote sensing products, and crops vary across the landscape. Current crop models and remote sensing products are insufficient for operational forecasting in African smallholder settings, where low crop yields can have disastrous outcomes for food security. Advances in data assimilation techniques for modeling these environments could significantly improve forecast skill in areas where advance warning of crop shortages is needed most.
Agricultural modelers use an understanding of how plants interact with the environment to estimate crop yields prior to harvest. Historical relationships between environmental variables and plant growth are used to fit statistical crop models, with biophysical variables such as soil moisture as model inputs. Forecast uncertainty is related to both model error and climate uncertainty. Seasonal climate models can be coupled with crop models to reduce climate uncertainty in crop yields. One method for reducing crop model error is via data assimilation, or the incorporation of new observations into the model at a given time step to correct variables describing the state of the crop system.
Satellite remote sensing can provide crop and soil measurements that can’t reasonably be collected in the field, and can be particularly valuable in areas with low on-the-ground monitoring capacity. IRI and JPL researchers tested the performance of crop models in large cropping areas run both with and without assimilation of remote sensing observations. In this case, researchers tested the daily assimilation of soil moisture and weekly assimilation of Leaf Area Index, which provides an estimate of how much vegetation cover is in a given area. Data assimilation produced major reductions in forecast uncertainty 1-3 months before harvest.
This technique was effective in a large-scale cropping environment, but further advances are needed for application in smallholder farming environments. A joint workshop with CCAFS and the Joint Research Centre in Ispra, Italy (JPL) was held in June 2012 to identify opportunities for improving crop forecasting in smallholder environments and promote collaboration across the data assimilation and crop forecasting research communities. Supported by CCAFS, JPL and IRI are continuing work on remote sensing applications to address the challenges of crop forecasting in developing countries, particularly in downscaling soil moisture data, and extracting vegetation signatures at sub-pixel scales. These advances can improve forecast skill on field and regional scales relevant to both small-scale crop management decisions and larger-scale policy processes in support of reducing climate risk in food systems.
Download the final report from the June 2012 Workshop: Combining Crop Models and Remote Sensing for Yield Prediction: Concepts, Applications and Challenges for Heterogeneous Smallholder Environments